AI talks turned the table and become more pessimistic
It is just another correction of exorbitant optimism and realisation of AI's current capabilities
AI can only help us to replace jobs in low noise data
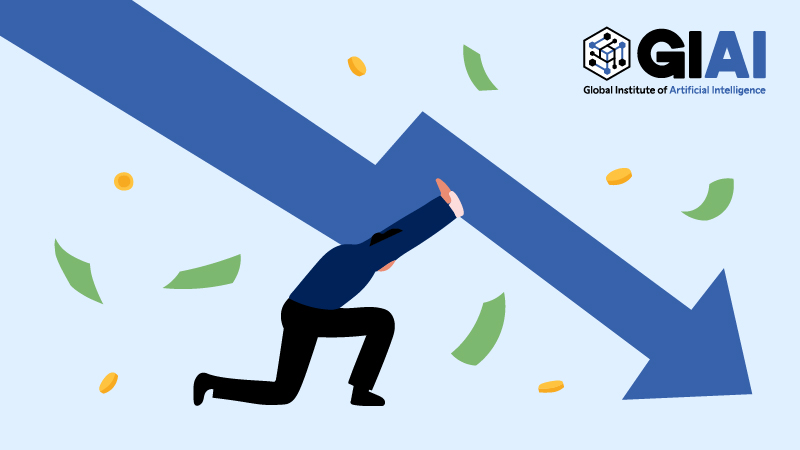
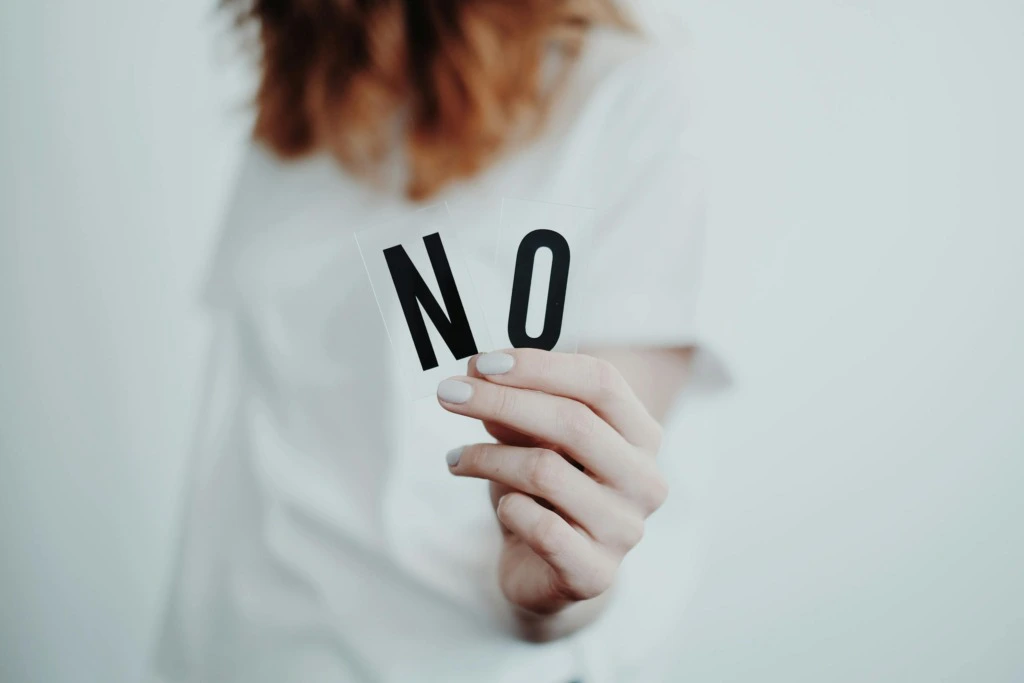
Top brains in AI/Data Science are driven to challenging jobs like modeling
Seldom a 2nd-tier company, with countless malpractices, can meet the expectations
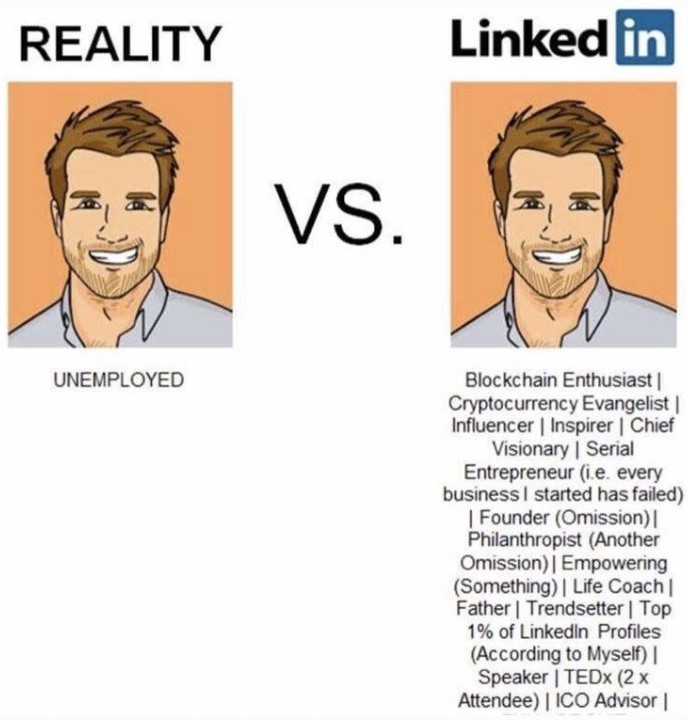
People following AI hype are mostly completely misinformed
AI/Data Science is still limited to statistical methods
Hype can only attract ignorance
As a professor of AI/Data Science, I from time to time receive emails from a bunch of hyped followers claiming what they call 'recent AI' can solve things that I have been pessimistic. They usually think 'recent AI' is close to 'Artificial General Intelligence', which means the program learns by itself and it is beyond human intelligence level.
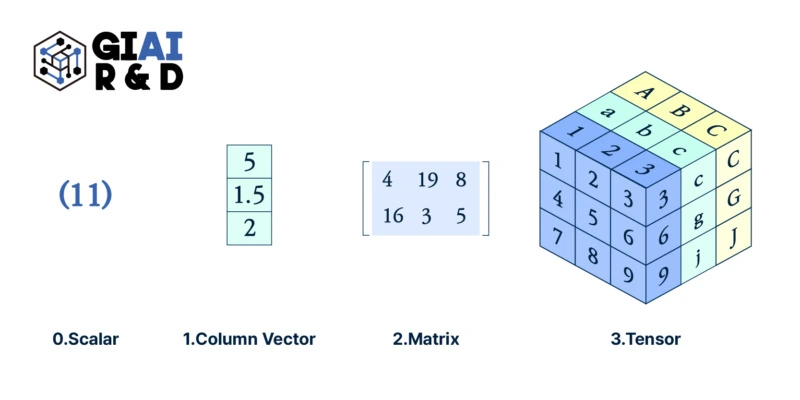
Transition from column to matrix, matrix to tensor as a baseline of data feeding changed the scope of data science,
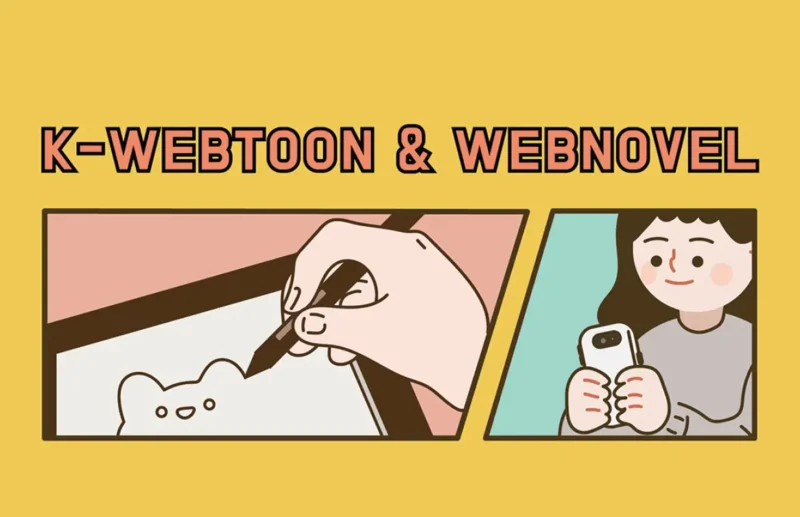
Web novel to Webtoon conversion is not only based on 'profitability'
If the novel author is endowed with money or bargaining power, 'Webtoonization' may be nothing more than a marketting tool for the web novel.
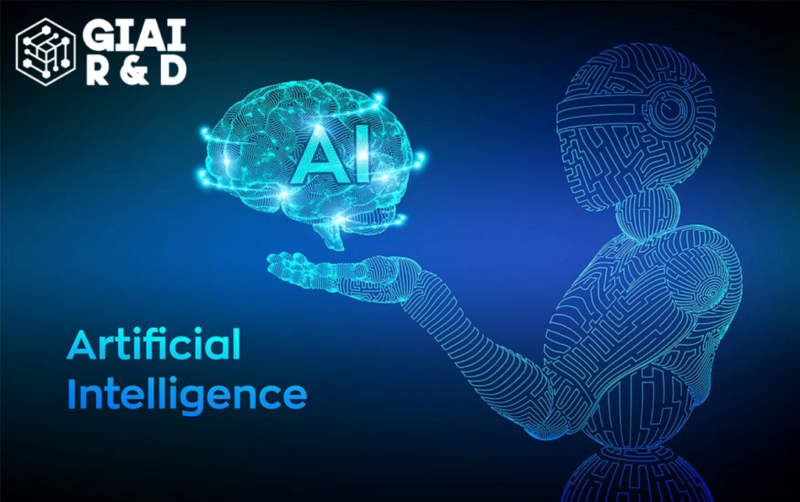
Asian companies convert degrees into years of work experience
Without adding extra values to AI degree, it doesn't help much in salary
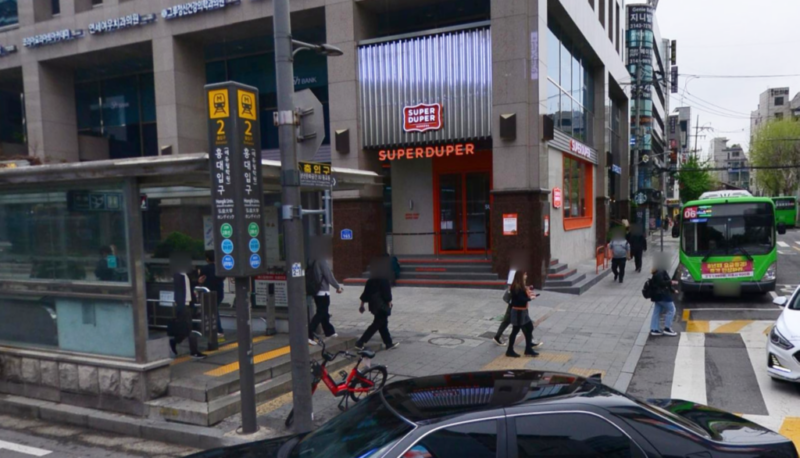
The relationship between a commercial district and the concentration of consumers in a specific generation mostly is not by causal effect
Simultaneity oftern requires instrumental variables
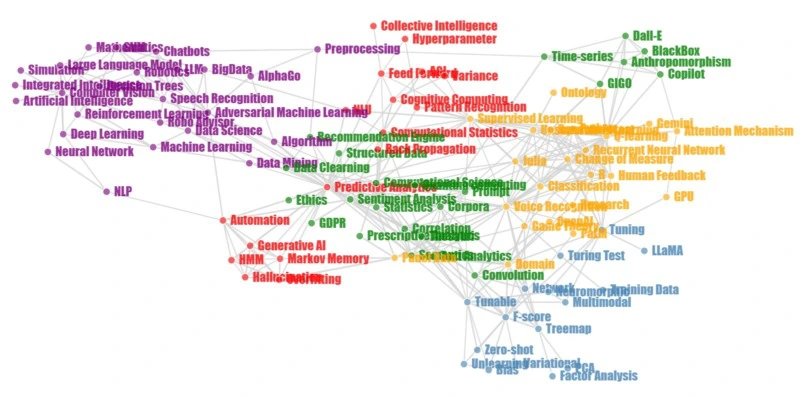
One-variable analysis can lead to big errors, so you must always understand complex relationships between various variables.
Data science is a model research project that finds complex relationships between various variables.
Obsessing with one variable is a past way of thinking, and you need to improve your way of thinking in line with the era of big data.
When providing data science speeches, when employees come in with wrong conclusions, or when I give external lectures, the point I always emphasize is not to do 'one-variable regression.'
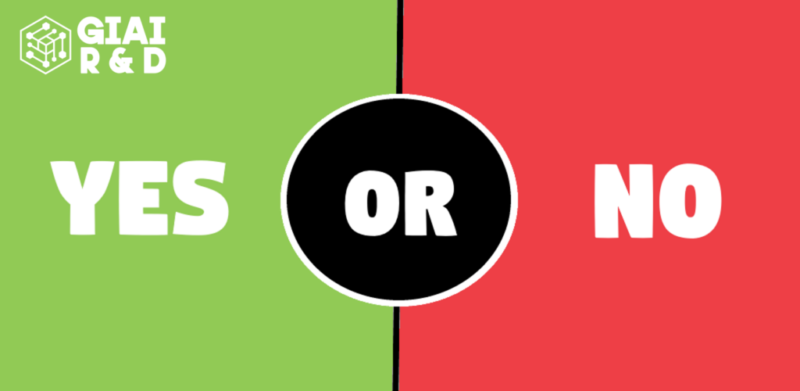
With high variance, 0/1 hardly yields a decent model, let alone with new set of data
What is known as 'interpretable' AI is no more than basic statistics