입력
수정
Boot camp is for software programming without mathematical training
MSc is a track for PhD, with in-depth scientific research written in the language of math and stat
We respect programmers, but our works are significantly varying
Due to the fact that we are running SIAI, an higher educational institution for AI/Data Science, we often have questions about the difference between Boot Camps for AI and MSc programmes. The shortest answer is the difference in Math requirements. Masters track is for people looking for academic training so that one can read academic papers in that subject. With PhD in the topic, we expect the student to be able to lead a research. From Boot Camp, sorry to be a little aggressive here, but we only expect a 'Coding Monkey'.
We are aware that many countries are shallow in AI/Data Science that they want employees only to be able to best use of Open AI's and AWS's libraries by Rest API. For that, boot camp should be enough, unless the boot camp teacher does not know how to do so. There are nearly infinite amount of contents for how to use Rest API for your software, regardless of your backend platform, be it an easy script languages like Python or tough functional ones like OCaml. Difficulties are not always indicators of determinants in challenges, and we, as data scientists at GIAI, care less about what language you use. What's important is how flexible your thinking for mathematically contained modeling.
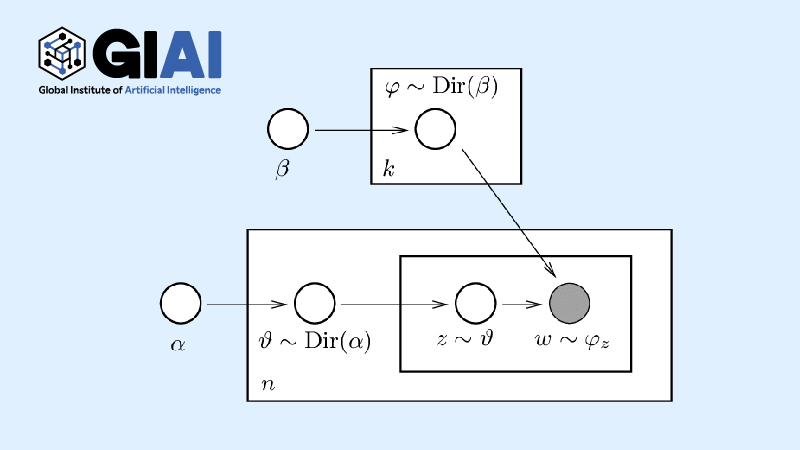
Boot camp for software programing, MSc for scientific training
Unfortunately, unless you are lucky enough to be born as smart as Mr. Ramanujan, you cannot learn math modeling skills from a bunch of blogs. Programming, however, has infinitely many proven records of excellent programmers without school traninng. Elon Musk is just one example. He did Economics and Physics in his undergrad at U Penn, and he only stayed one day in the mechanical engineering PhD program at Stanford University. Programming is nothing more than a logic, but math needs too many building blocks to understand the language.
When we first build SIAI, we had quite a lengthy discussion for weeks. Keith was firm that we should stick to mathematical aspects of AI/Data Science. (which doesn't mean we should only teach math, just to avoid any misunderstanding.) Mc wanted two tier tracks for math and coding. We later found that with coding, it is unlikely that we can have the school accreditted by official parties, so we end up with Keith's idea. Besides, we have seen too many Boot Camps around the world that we do not believe we can be competitive in that regard.
The founding motto of the school is 'Rerum Cognoscere Causaus', meaning 'the real cause of things'. With mathematical tools, we were sure that we can teach what are the reason behind a computational model was first introduced. Indeed, Keith has done so well in his Scientific Programming that most students no longer bound to media brainwashing that Neural Network is the most superior model.
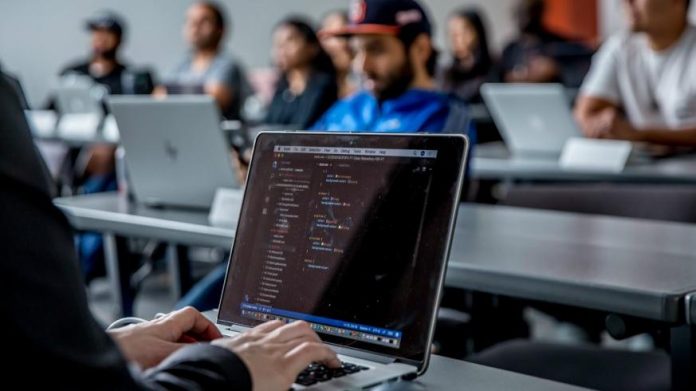
Scientists do our own stuff
If you just go through Boot Camps for coding, chances are that you can learn the limitations of Neural Network just by endless trials and errors, if not somebody's Medium posts and Reddit comments. In other words, without the proper math training, it is unlikely one can understand how the computational logics of each model are built, which makes us to aloof from all programmers without necessary math training.
The very idea comes from multiple rounds of uneasy exposures to software engineers without a shred of understanding in modeling side of AI. They usually claim that Neural Network is proven to be the best model, and they do not need any other model knowledge. And all they have to do is to run and test it. Researchers at GIAI are trained scientists, and we mostly can guess what will happen just by looking at equations. And, most importantly, we are well aware that NN is the best model only for certain tasks.
They kept claim that they were like us, and some of them wanted to build a formal assocation with SIAI (and later GIAI). It's hard for us to work with them, if they keep that attitude. These days, whenever we are approached by third parties, if they want to be at equals with us, we ask them to show us math training levels. Please make no mistake that we respect them as software engineers, but we do not respect them as scientists.
Guess aforementioned story and internal discomfort tells you the difference between software engineers and data/research scientists, let alone tools that we rely on.
We screen out students by admission exams in math/stat
With the experience, Keith initiated two admission exams for our MSc AI/Data Science programmes. At the very beginning, we thought there will be plenty of qualifying students, so we used final year undergrad materials. There was a disaster. We gave them two months of dedicated training. Provided similar exams and solved each one of them with extra detail. But, only 2 out of 30 students were able to get grades good enough to be admitted.
We lowered the level down to European 2nd year (perhaps American 3rd year), and the outcome wasn't that different. Students were barely able to grasp superficial concepts of key math/stat. This is why we were kinda forced to create an MBA program that covers European 2nd year teaching materials with ample amount of business application cases. With that, students survive, but answer keys in their final exam tell us that many of them belong to coding Boot Camps, not SIAI.
From year 2025 and onwards, we will have one admission exam for MSc AI/Data Science (2 year) in March, after 2 months pre-training in Jan and Feb. The exam materials will be 2nd year undergrad level. If a student passes, we offer an exam with one notch up in June, again after 2 months pre-training in Apr and May. This will give them MSc AI/Data Science (1 year) admission.
Students who failed the 2-year track admission, we offer them MBA AI program admission, which covers some part of the 2-year track courses. If they think they are ready, then in the following year, they can take the admission exam again. After a year of various courework, some students have shown better performance, based on our statistics, but not by much. It seemed like the brain has its limit that they cannot go above.
Precisely by the same reason, we are reasonably sure that not that many applicants will be able to come to 2-year track, and almost no one for the 1-year track. More details are available from below link: